Hi there! I’ve been an active member of this community for a while -- first as a PX customer, then as a PX Client Outcomes Manager, and now as a Gainsight Digital Led CS Ops Specialist (translation: I’m using our PX and CS products to drive our Digital Led program at Gainsight).
Now that I’m helping us drink our own champagne, I want to share my learnings with you and hopefully learn from you as well. Feel free to comment below with any “So how does Gainsight do x, y, and z?” topics you want to hear more about!
Now, onto the topic at hand. Have you ever fallen down the analytics rabbit hole? I know I have.
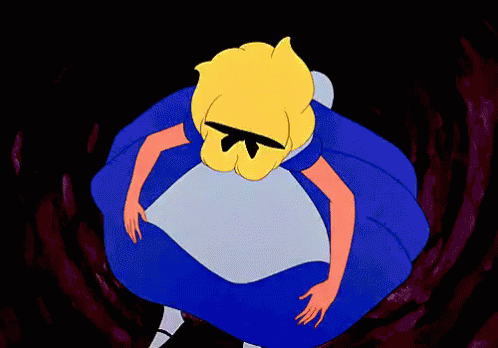
I look at a super swanky PX feature adoption report, then I wonder --
What about new users?
How does the customer segment affect this report?
What about a new admin at a longtime enterprise customer who is using our latest flagship feature?
What about…?
You get the idea. Of course, it’s not bad to ask these questions. However, to avoid falling down the rabbit hole, I am learning to conduct 1) data discovery sessions, 2) objective-driven analysis, and 3) cyclical batch analysis.
1 - Data discovery sessions
It can be helpful to have data discovery sessions where you simply follow your curiosity without an exact objective in mind.
This kind of session can help you:
-
Discover patterns you may not have thought to look for
-
Understand how the data in your instance is structured
-
Discover any caveats or data inconsistencies to resolve or keep in mind for future analysis
-
Have a more holistic understanding of your data, which could come in handy during future, more objective-driven analysis
That said, what I’ve learned is to keep these sessions time bound, otherwise I will surely discover myself fully down the rabbit hole.
2 - Objective-driven analysis
On the other hand, conducting objective-driven analysis means I am looking to take an action or make a decision based on the conclusions I draw from the data.
The process below (which came out of a conversation with a PX customer) is especially helpful to ensure that the analysis you’re about to do really is objective-driven.
- Determine the question to answer.
- If the answer to that question is “no” or “lower xx than we thought”, do you know what action you will take?
- If the answer to that question is “yes” or “higher xx than we thought”, do you know what action you will take?
- If you do not have viable answers for 2 and 3, then the analysis is not objective-driven (may be worth considering during a time of data discovery). If there are good answers to 2 and 3, the analysis effort is objective-driven.
With this approach, I can focus my time on questions that could actually steer the ship, so to speak.
3 - Cyclical batch analysis
(...what does that term even mean? It’s pretty self explanatory, it just sounds fancy.)
Finally, I find it helpful to do a “batch analysis” once/month or once/quarter for questions like “How effective are our onboarding engagements at increasing user adoption and retention?”
If I attempted to answer that question every time I launched or edited an engagement, I would almost certainly fall down the rabbit hole. By taking a bird’s eye view on a cyclical basis, I can 1) save myself time by batching similar tasks and 2) make improvements all at once, rather than piecemeal.
To sum up, the key to avoid falling down the analytics rabbit hole is to have guardrails.
What do you think? Are there any other ways you avoid the rabbit hole? Asking for a friend